Recommendation Engine
Recommendation engines help companies recommend the right product or service to their customers +Show More
Products | Position | Customer satisfaction | |||
---|---|---|---|---|---|
|
Leader
|
Satisfactory
|
|
||
Insider—one platform for individualized, cross-channel experiences—enables enterprise marketers to connect customer data across channels and systems, predict their future behavior with an AI intent engine, and orchestrate individualized experiences to customers. Marketers use Insider’s platform to deliver experiences across channels like Web, App, Web Push, Email, SMS, Messaging Apps (WhatsApp, Facebook Messenger, RCS), Ads, and more. Insider is trusted by over 1000 global businesses, including IKEA, Adidas, Estee Lauder, MAC Cosmetics, Singapore Airlines, Burger King, Virgin, Toyota, New Balance, Samsung, Newsweek, MediaMarkt, Nissan, AVIS, Marks & Spencer, Allianz, BBVA, Dominos, Avon, and CNN. Insider has been recognized as the #1 Leader everywhere by analysts. Insider was featured as a Leader in the Magic Quadrant for Personalization Engines 2021 by Gartner, Leader in the Wave for Cross-Channel Campaign Management 2021 by Forrester, and a Leader in Marketscape for Worldwide Customer Data Platforms 2021 by IDC. The company has been named the #1 Leader on G2’s Mobile Marketing Software, Customer Data Platforms, and Personalization Grids, with a 4.7/5 rating based on customer reviews and recognized as the Top 50 best software products in the world by G2. Recently, Insider unlocked its unicorn status after announcing its $121 Million Series D funding round, led by Riverwood Capital and joined by Sequoia, Wamda, and Endeavor Catalyst. CrunchBase ranked Insider’s co-founder and CEO Hande Cilingir as one of the top women CEOs outside the US. Insider has 25 offices in London, Paris, Singapore, Tokyo, Hong Kong, Seoul, Sydney, Indianapolis, New York, São Paulo, Helsinki, Barcelona, Dubai, Moscow, Warsaw, Taipei, Jakarta, Manila, Istanbul, Kiev, Ho Chi Minh City, Bangkok, Amsterdam, Ankara, and Kuala Lumpur.
Basis for EvaluationWe made these evaluations based on the following parameters; Customer satisfaction
Average rating
Market presence
Number of case studies
100-200 case studies
Company's number of employees
1k-2k employees
Company's social media followers
100k-1m followers
Total funding
$250-500m
# of funding rounds
8
Latest funding date
May 24, 2023
Last funding amount
$100-250m
Company
Type of company
private
Founding year
2012
|
|||||
|
Leader
|
Satisfactory
|
|
||
Braze is a leading comprehensive customer engagement platform that powers interactions between consumers and brands they love. With Braze, global brands can ingest and process customer data in real time, orchestrate and optimize contextually relevant, cross-channel marketing campaigns and continuously evolve their customer engagement strategies. Braze has been recognized as one of Fortune’s 2022 Best Workplaces in New York, Fortune’s 2021 Best Workplace for Millennials, and 2021 UK Best Workplaces for Women by Great Place to Work. The company is headquartered in New York with offices in Austin, Berlin, Chicago, London, Paris, San Francisco, Singapore, and Tokyo. Learn more at braze.com.
Basis for EvaluationWe made these evaluations based on the following parameters; Customer satisfaction
Average rating
Market presence
Number of case studies
50-100 case studies
Company's number of employees
1k-2k employees
Company's social media followers
100k-1m followers
Total funding
$100-250m
# of funding rounds
9
Latest funding date
June 21, 2023
Company
Type of company
public
Founding year
2011
|
|||||
|
Leader
|
Satisfactory
|
|
||
Optimizely is the world's leading experimentation platform, empowering marketing and product teams to test, learn and deploy winning digital experiences
Basis for EvaluationWe made these evaluations based on the following parameters; Customer satisfaction
Average rating
Market presence
Number of case studies
200-300 case studies
Company's number of employees
200-300 employees
Company's social media followers
50k-100k followers
Total funding
$250-500m
# of funding rounds
9
Latest funding date
June 18, 2019
Last funding amount
$50-100m
Company
Type of company
private
Founding year
2010
|
|||||
|
Leader
|
Satisfactory
|
|
||
Bloomreach offers an open, java CMS and an AI-powered search and merchandising solution designed for businesses to boost conversions
Basis for EvaluationWe made these evaluations based on the following parameters; Customer satisfaction
Average rating
Market presence
Number of case studies
50-100 case studies
Company's number of employees
1k-2k employees
Company's social media followers
50k-100k followers
Total funding
$250-500m
# of funding rounds
7
Latest funding date
October 4, 2022
Last funding amount
$10-50m
Company
Type of company
private
Founding year
2009
|
|||||
|
Leader
|
Satisfactory
|
|
||
Dotdigital is a cross-channel marketing automation platform that helps digital marketers and developers deliver communications across the customer journey. We harness the power of customer data, powering engagement, conversion, and loyalty for brands as they grow and scale. Customers love our easy-to-use platform that connects first-party data across the systems, surfacing powerful insights and automating predictive cross-channel messages. Dotdigital’s 400+ employees serve mid-market and enterprise companies around the world and across industries. We aspire to inspire responsible marketing and are committed to sustainability, privacy & security. Dotdigital is proud to be the world’s first carbon-neutral, ISO14001, ISO 27701 & ISO27001 certified marketing automation platform.
Basis for EvaluationWe made these evaluations based on the following parameters; Customer satisfaction
Average rating
Market presence
Company's number of employees
400-1k employees
Company's social media followers
50k-100k followers
|
|||||
|
Challenger
|
Satisfactory
|
|
||
Basis for EvaluationWe made these evaluations based on the following parameters; Customer satisfaction
Average rating
Market presence
Number of case studies
30-40 case studies
Company's number of employees
400-1k employees
Company's social media followers
30k-40k followers
Total funding
$250-500m
# of funding rounds
7
Latest funding date
June 15, 2021
Last funding amount
$100-250m
Company
Type of company
private
Founding year
2013
|
|||||
|
Challenger
|
Satisfactory
|
|
||
CleverTap is the all-in-one engagement platform that helps brands unlock limitless customer lifetime value by helping them create personalized experiences to retain their most valuable customers. The platform empowers businesses to orchestrate experiences for individuals across their lifecycles and design personalized journeys that span a lifetime. It offers analytics that encompasses every aspect of the lifecycle, enabling businesses to measure and optimize each experience in real time. Its unique AI capability is insightful, empathetic, and prescriptive, facilitating smarter and faster decisions. The all-in-one platform unifies experiences from every touchpoint, paving the way for a new era of customer engagement. The platform is powered by TesseractDB™ – the world’s first purpose-built database for customer engagement, offering both speed and economies of scale. CleverTap is trusted by 2000 customers, including Electronic Arts, TiltingPoint, Gamebasics, Big Fish, MobilityWare, TED, English Premier League, TD Bank, Carousell, AirAsia, Papa John’s, and Tesco. Backed by leading investors such as Peak XV Partners, Tiger Global, Accel, CDPQ and 360 One, the company is headquartered in Mountain View, California, with presence in San Francisco, New York, São Paulo, Bogota, London, Amsterdam, Sofia, Dubai, Mumbai, Bangalore, Singapore, and Jakarta. For more information, visit clevertap.com or follow us on: LinkedIn: https://www.linkedin.com/company/clevertap/ Twitter: https://twitter.com/CleverTap
Basis for EvaluationWe made these evaluations based on the following parameters; Customer satisfaction
Average rating
Market presence
Number of case studies
50-100 case studies
Company's number of employees
400-1k employees
Company's social media followers
50k-100k followers
Total funding
$100-250m
# of funding rounds
6
Latest funding date
August 9, 2022
Last funding amount
$100-250m
Company
Type of company
private
Founding year
2013
|
|||||
|
Challenger
|
Satisfactory
|
|
||
Emarsys, now an SAP company, empowers digital marketing leaders and business owners with the only omnichannel customer engagement platform built to accelerate business outcomes. By rapidly aligning desired business results with proven omnichannel customer engagement strategies — crowdsourced from leading brands across your industry — our platform enables you to accelerate time to value, deliver superior one-on-one experiences and produce measurable results... fast. Emarsys is the platform of choice for more than 1,500 customers around the world. Join thousands of leading brands who trust Emarsys to deliver the predictable, profitable outcomes that their businesses demand and the highly personalized omnichannel experiences that their customers deserve. For more information, visit www.emarsys.com
Basis for EvaluationWe made these evaluations based on the following parameters; Customer satisfaction
Average rating
Market presence
Number of case studies
40-50 case studies
Company's number of employees
400-1k employees
Company's social media followers
50k-100k followers
Total funding
$50-100m
# of funding rounds
2
Latest funding date
October 31, 2016
Last funding amount
$10-50m
Company
Type of company
private
Founding year
2000
|
|||||
|
Challenger
|
Satisfactory
|
|
||
Dynamic Yield's Personalization Anywhere tech helps marketers increase revenue by individualizing each user's interactions across web, mobile and email.
Basis for EvaluationWe made these evaluations based on the following parameters; Customer satisfaction
Average rating
Market presence
Number of case studies
30-40 case studies
Company's number of employees
400-1k employees
Company's social media followers
30k-40k followers
Total funding
$100-250m
# of funding rounds
6
Latest funding date
November 2, 2018
Last funding amount
$10-50m
Company
Type of company
private
Founding year
2011
|
|||||
|
Challenger
|
Satisfactory
|
|
||
Evergage's real-time personalization and customer data platform (CDP) enables companies to leverage behavioral analytics and machine learning
Basis for EvaluationWe made these evaluations based on the following parameters; Customer satisfaction
Average rating
Market presence
Company's number of employees
50k-100k employees
Company's social media followers
5m-6m followers
|
“-”: AIMultiple team has not yet verified that vendor provides the specified feature. AIMultiple team focuses on feature verification for top 10 vendors.
Sources
AIMultiple uses these data sources for ranking solutions and awarding badges in recommendation engines:
Recommendation Engine Leaders
According to the weighted combination of 4 metrics
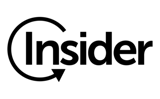
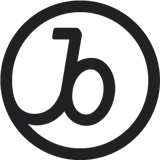
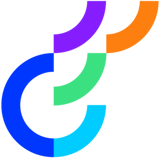
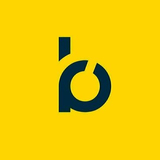
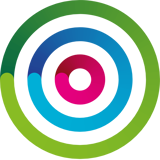
What are recommendation engine
customer satisfaction leaders?
Taking into account the latest metrics outlined below, these are the current recommendation engine customer satisfaction leaders:
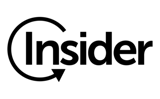
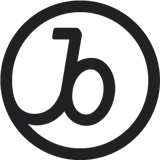
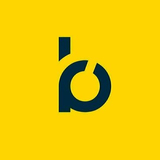
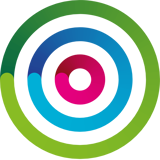
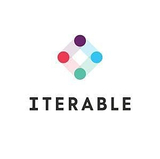
Which recommendation engine solution provides the most customer satisfaction?
AIMultiple uses product and service reviews from multiple review platforms in determining customer satisfaction.
While deciding a product's level of customer satisfaction, AIMultiple takes into account its number of reviews, how reviewers rate it and the recency of reviews.
- Number of reviews is important because it is easier to get a small number of high ratings than a high number of them.
- Recency is important as products are always evolving.
- Reviews older than 5 years are not taken into consideration
- older than 12 months have reduced impact in average ratings in line with their date of publishing.
What are recommendation engine
market leaders?
Taking into account the latest metrics outlined below, these are the current recommendation engine market leaders:
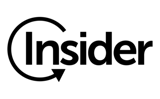
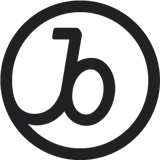
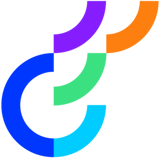
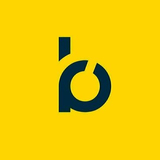
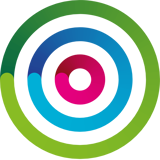
Which Recommendation Engine products published the most case studies?
We analyzed 53 Recommendation Engine case studies and found that these products are the top contributors:
- Dynamic Yield
- Adobe Target
- Vue.ai
Which one has collected the most reviews?
AIMultiple uses multiple datapoints in identifying market leaders:
- Product line revenue (when available)
- Number of reviews
- Number of case studies
- Number and experience of employees
- Social media presence and engagement
What are the most mature recommendation engines?
Which one has the most employees?
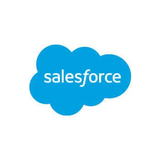
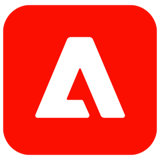
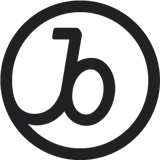
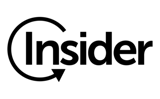
Which recommendation engine companies have the most employees?
427 employees work for a typical company in this solution category which is 404 more than the number of employees for a typical company in the average solution category.
In most cases, companies need at least 10 employees to serve other businesses with a proven tech product or service. 20 companies with >10 employees are offering recommendation engines. Top 3 products are developed by companies with a total of 400k employees. The largest company in this domain is IBM with more than 300,000 employees. IBM provides the recommendation engine solution: IBM Watson Real-Time Personalization
Insights
What are the most common words describing recommendation engines?
This data is collected from customer reviews for all recommendation engine companies. The most positive word describing recommendation engines is “Easy to use” that is used in 6% of the reviews. The most negative one is “Difficult” with which is used in 2% of all the recommendation engine reviews.
What is the average customer size?
According to customer reviews, most common company size for recommendation engine customers is 51-1,000 employees. Customers with 51-1,000 employees make up 51% of recommendation engine customers. For an average E-Commerce solution, customers with 51-1,000 employees make up 37% of total customers.
Customer Evaluation
These scores are the average scores collected from customer reviews for all recommendation engines. Recommendation Engines are most positively evaluated in terms of "Overall" but falls behind in "Likelihood to Recommend".
What are the benefits of Recommendation Engine?
The most commonly cited benefits of Recommendation Engine are:
- Improved customer experience
- Increased sales
- Increased customer engagement
- Time saving
- Scalability
Discover all Recommendation Engine benefits
Where are recommendation engine vendors' HQs located?
Trends
What is the level of interest in recommendation engines?
This category was searched on average for 3.5k times per month on search engines in 2024. This number has decreased to 0 in 2025. If we compare with other e-commerce solutions, a typical solution was searched 278 times in 2024 and this decreased to 0 in 2025.
Learn more about Recommendation Engines
Recommendation engines are generally used to boost sales processes along with the relationship between the organization and customers. To learn all benefits, check out our article.
Challenges include:
- Cold start problem: What should you recommend to new users? Should you recommend the most commonly recommended items or should you try to understand more about the user? Answers to such questions depend on the specific application.
- Obvious recommendations: With no long tail data, recommendation systems make quite obvious recommendations which could easily be programmed by a few rules. Data is crucial for a recommendation system.
- Static recommendations that become outdated with changing tastes: If the system is not continously learning, such a scenario is inevitable. Companies are advised to invest in continuously learning systems
- Recommendations that violate personal privacy: Consumption data is personal data and using such data for recommendations requires care even when the recommendation is only shared by the user. A NY Times article from 2012 includes an anecdote about how Target predicted a teen's pregnancy before her father. Though we don't know if such a thing really happened, it is indeed an example of how innocent looking recommendations can violate personal privacy.
Recommendation engines have three basic steps to make recommendations:
Data Collection
Core of a recommendation engine is consumer data. These engines collect implicit and explicit data.
- Implicit data is the information that is gathered unintentionally from customers by checking their website history. Examples are web search history, clicks and order history.
- Explicit data is created by customers’ inputs such as ratings and likes/dislikes.
Data storage
As the amount of data you store increases, you provide better recommendations for your customers. Organizations need to keep as much data as possible on the cloud or enterprise to analyze customers and divide them into segments.
Data Analysis and Recommendation
Recommendation engines analyze data by filtering it to extract relevant insights to make the final recommendations.
Recommendation systems can be useful and applicable to various industries in the B2C environment. We’ve explained those use cases before, feel free to check our article.
Recommendations depend on a combination of similar users' actions (collaborative filtering), products similar to those consumed by the user (content based filtering) or the context of the user (context aware filtering):
Content-based filtering
Content based filtering, as its name refers, is recommending a product that is similar to products the customer liked before. Below is an example of a movie recommendation content based filtering. Rabin is a user who mostly watches commercial dram movies and the system provides Movie A and Movie B as a recommendation. The downside of content-based filtering is product mappings are manual and depend on labelers’ bias.
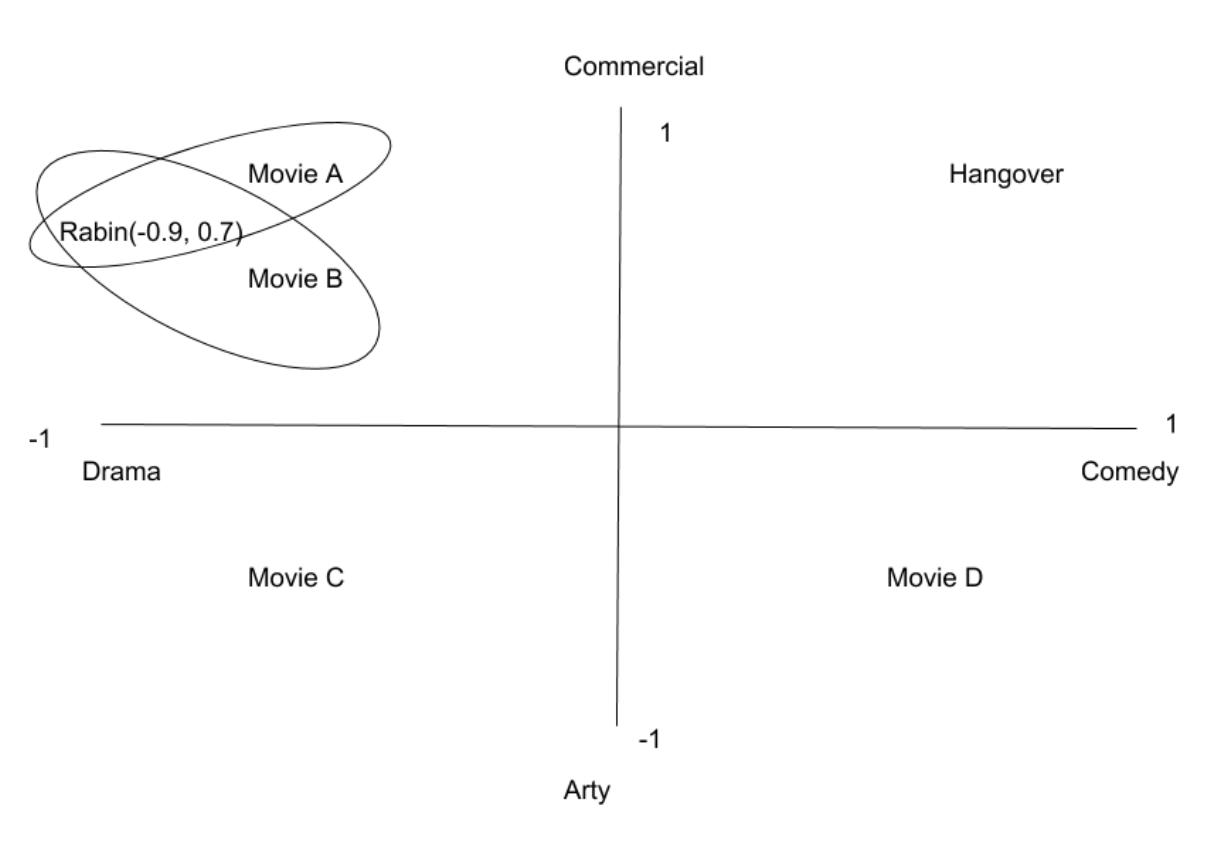
Source:Medium
Another example is if the user rated a song from an artist, system recommends him another song from the same album.
Collaborative filtering
Collaborative filtering methods are divided into two categories:
- User-based collaborative filtering: Engine recommends a product if the product has been liked by users similar to the user.
- Item-based collaborative filtering: Based on users’ previous ratings, system identifies similar items. For example, if users A,B and C rated books X and Y, then when a new user purchases book Y, systems recommend purchasing book X as well due to the pattern created by A,B and C users.
Context aware filtering adjusts recommendations based on the time, place, the users' consumption right before the recommendation. For example, ice creams should be recommended more often in summer.
As the competition in all industries is increasing, keeping their customers engaged is an important goal for organizations. Recommendation engines enable organizations to increase their sales by upselling (selling a higher volume of products that they buy) or cross-selling (selling new products) to existing customers. Here are some recommendation engine examples from tech leaders:
- 35% of Amazon.com’s revenue is generated by its recommendation engine.
- 75% of users in Netflix choose movies/tv series according to recommendation engine suggestions. Netflix executives Carlos A. Gomez-Uribe and Neil Hunt state that recommendations reduce the churn rate by several percentage points. This increases the lifetime value of existing customers that’s why they believe recommendations save them more than $1B per year.
- Spotify first released Discover Weekly playlist recommendations in 2015 and they experienced an 80% revenue increase with 40 million Discover Weekly users(40% of total users by that time) in 2016.