Data science / ML / AI platform
AI platforms (also called machine learning platforms or data science platforms) allow users to analyze data and process data, build machine learning models, deploy and maintain these models +Show More
Products | Position | Customer satisfaction | |||
---|---|---|---|---|---|
|
Leader
|
Satisfactory
|
|
||
Alteryx is a leader in data science and self-service analytics with a platform that can prep, blend, enrich, and analyze data, manage and deploy predictive analytics solutions
Basis for EvaluationWe made these evaluations based on the following parameters; Customer satisfaction
Average rating
Market presence
Number of case studies
300-400 case studies
Company's number of employees
2k-3k employees
Company's social media followers
100k-1m followers
Total funding
$1-1bn
# of funding rounds
4
Latest funding date
February 28, 2023
Last funding amount
$250-500m
Company
Type of company
public
Founding year
2011
|
|||||
|
Leader
|
Satisfactory
|
|
||
Basis for EvaluationWe made these evaluations based on the following parameters; Customer satisfaction
Average rating
Market presence
Number of case studies
10-20 case studies
Company's number of employees
300-400 employees
Company's social media followers
100k-1m followers
Total funding
$100-250m
# of funding rounds
4
Latest funding date
June 17, 2022
Last funding amount
$1-5m
Company
Type of company
private
Founding year
2009
|
|||||
|
Leader
|
Satisfactory
|
|
||
Work smarter with artificial intelligence that's built right into Salesforce. Get more done with Einstein AI, your smart CRM assistant.
Basis for EvaluationWe made these evaluations based on the following parameters; Customer satisfaction
Average rating
Market presence
Company's number of employees
5-10 employees
Company's social media followers
5k-10k followers
Total funding
$50-100m
# of funding rounds
7
Latest funding date
October 18, 2022
Company
Type of company
public
Founding year
1999
|
|||||
|
Leader
|
Satisfactory
|
|
||
RapidMiner is a data science platform for teams that unites data prep, machine learning, and predictive model deployment.
Basis for EvaluationWe made these evaluations based on the following parameters; Customer satisfaction
Average rating
Market presence
Number of case studies
30-40 case studies
Company's number of employees
30-40 employees
Company's social media followers
30k-40k followers
Total funding
$10-50m
# of funding rounds
4
Latest funding date
October 2, 2020
Last funding amount
$1-5m
Company
Type of company
private
Founding year
2007
|
|||||
|
Leader
|
Satisfactory
|
|
||
The fastest annotation platform and services for training AI.
A complete set of solutions for image and video annotation and an annotation service with integrated tooling, on-demand narrow expertise in various fields, and a custom neural network, automation, and training models powered by AI.
Basis for EvaluationWe made these evaluations based on the following parameters; Customer satisfaction
Average rating
Market presence
Number of case studies
5-10 case studies
Company's number of employees
200-300 employees
Company's social media followers
10k-20k followers
Total funding
$10-50m
# of funding rounds
5
Latest funding date
December 28, 2023
Last funding amount
$5-10m
Company
Type of company
private
Founding year
2019
|
|||||
|
Challenger
|
Satisfactory
|
|
||
TensorFlow is an open source software library for numerical computation using data flow graphs.
Basis for EvaluationWe made these evaluations based on the following parameters; Customer satisfaction
Average rating
Market presence
Company's number of employees
100k-1m employees
Company's social media followers
30m-40m followers
Company
Type of company
private
Founding year
2017
|
|||||
|
Challenger
|
Satisfactory
|
|
||
Together with IBM Watson Machine Learning, IBM Watson Studio is a leading data science and machine learning platform
Basis for EvaluationWe made these evaluations based on the following parameters; Customer satisfaction
Average rating
Market presence
Company's social media followers
100k-1m followers
# of funding rounds
0
Company
Type of company
public
Founding year
1911
|
|||||
|
Challenger
|
Satisfactory
|
|
||
With more than 30 million users, Anaconda is the world’s most popular data science platform and the foundation of modern machine learning. We pioneered the use of Python for data science, champion its vibrant community, and continue to steward open-source projects that make tomorrow’s innovations possible. Our enterprise-grade solutions enable corporate, research, and academic institutions around the world to harness the power of open-source for competitive advantage, groundbreaking research, and a better world.
Basis for EvaluationWe made these evaluations based on the following parameters; Customer satisfaction
Average rating
Market presence
Number of case studies
1-5 case studies
Company's number of employees
300-400 employees
Company's social media followers
100k-1m followers
Total funding
$50-100m
# of funding rounds
6
Latest funding date
April 13, 2021
Last funding amount
$10-50m
Company
Type of company
private
Founding year
2012
|
|||||
|
Challenger
|
Satisfactory
|
|
||
Use powerful data mining software, SAS Enterprise Miner, to create accurate predictive and descriptive models for large volumes of data.
Basis for EvaluationWe made these evaluations based on the following parameters; Customer satisfaction
Average rating
Market presence
Company's number of employees
10k-20k employees
Company's social media followers
100k-1m followers
Total funding
$1-5m
# of funding rounds
2
Latest funding date
November 8, 2022
Last funding amount
$1-5m
Company
Type of company
private
Founding year
1976
|
|||||
|
Challenger
|
Satisfactory
|
|
||
IBM SPSS Modeler provides predictive analytics to help you uncover data patterns, gain predictive accuracy and improve decision making
Basis for EvaluationWe made these evaluations based on the following parameters; Customer satisfaction
Average rating
Market presence
Company's social media followers
100k-1m followers
# of funding rounds
0
Company
Type of company
public
Founding year
1911
|
“-”: AIMultiple team has not yet verified that vendor provides the specified feature. AIMultiple team focuses on feature verification for top 10 vendors.
Sources
AIMultiple uses these data sources for ranking solutions and awarding badges in data science / ML / AI platforms:
Data science / ML / AI Leaders
According to the weighted combination of 4 metrics
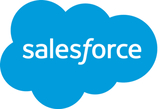
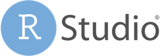
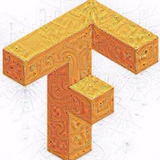
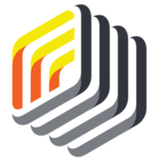
What are data science / ML / AI
customer satisfaction leaders?
Taking into account the latest metrics outlined below, these are the current data science / ML / AI customer satisfaction leaders:
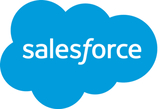
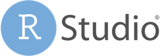
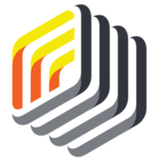
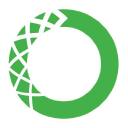
Which data science / ML / AI solution provides the most customer satisfaction?
AIMultiple uses product and service reviews from multiple review platforms in determining customer satisfaction.
While deciding a product's level of customer satisfaction, AIMultiple takes into account its number of reviews, how reviewers rate it and the recency of reviews.
- Number of reviews is important because it is easier to get a small number of high ratings than a high number of them.
- Recency is important as products are always evolving.
- Reviews older than 5 years are not taken into consideration
- older than 12 months have reduced impact in average ratings in line with their date of publishing.
What are data science / ML / AI
market leaders?
Taking into account the latest metrics outlined below, these are the current data science / ML / AI market leaders:
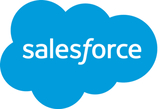
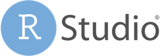
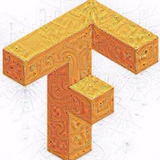
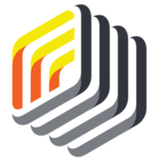
Which one has collected the most reviews?
AIMultiple uses multiple datapoints in identifying market leaders:
- Product line revenue (when available)
- Number of reviews
- Number of case studies
- Number and experience of employees
- Social media presence and engagement
What are the most mature data science / ML / AI platforms?
Which one has the most employees?
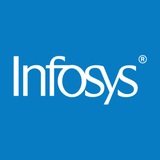
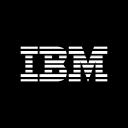
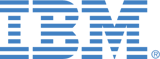
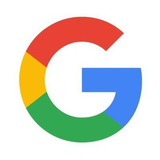
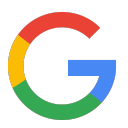
Which data science / ML / AI companies have the most employees?
58 employees work for a typical company in this solution category which is 35 more than the number of employees for a typical company in the average solution category.
In most cases, companies need at least 10 employees to serve other businesses with a proven tech product or service. 104 companies with >10 employees are offering data science / ml / ai platforms. Top 3 products are developed by companies with a total of 900k employees. The largest company in this domain is EdgeVerve Systems with more than 300,000 employees. EdgeVerve Systems provides the data science / ML / AI solution: Infosys Nia
Insights
What are the most common words describing data science / ML / AI platforms?
This data is collected from customer reviews for all data science / ML / AI companies. The most positive word describing data science / ML / AI platforms is “Easy to use” that is used in 6% of the reviews. The most negative one is “Difficult” with which is used in 3% of all the data science / ML / AI reviews.
What is the average customer size?
According to customer reviews, most common company size for data science / ML / AI customers is 1,001+ employees. Customers with 1,001+ employees make up 37% of data science / ML / AI customers. For an average AI Solutions solution, customers with 1,001+ employees make up 31% of total customers.
Customer Evaluation
These scores are the average scores collected from customer reviews for all data science / ML / AI platforms. Data science / ML / AI platforms are most positively evaluated in terms of "Overall" but falls behind in "Ease of Use".
Where are data science / ML / AI vendors' HQs located?
Trends
What is the level of interest in data science / ML / AI platforms?
This category was searched on average for 14.9k times per month on search engines in 2024. This number has decreased to 0 in 2025. If we compare with other ai solutions solutions, a typical solution was searched 13.1k times in 2024 and this decreased to 0 in 2025.
Learn more about Data science / ML / AI platforms
We can define artificial intelligence (AI) as the machines that can mimic human intelligence to perform tasks and learn from them. These tasks require human capabilities like decision-making, visual perception, and speech recognition. You can read more about this in the related section of our in-depth AI guide.
AI platforms can be separated into three main layers for enabling businesses to deploy machine learning models from a broad range of frameworks, languages, platforms, and tools. These three layers are:
- Data and Integration
- Experimentation
- Operations and Deployment
You can read the related section of our AI platforms guide to learn more about these layers.
The evolution of AI platforms is highly connected to the future of AI. We are bullish about AI approaches becoming more accurate and effective due to the factors listed below. In addition, we expect AI platforms to further automate manual aspects of machine learning such as feature engineering by incorporating mature capabilities of auto ML software. Feel free to visit our AutoML vendor list, if you are interested.
- Advances in computing power: AI platforms will be able to handle more complicated machine learning models with advances in computing power. These advances include AI-powered chips, quantum computing, and intelligent GPUs.
- The growing amount of data: The amount of data available for businesses rapidly grows every day.
- Advances in algorithm design: With better algorithm designs, AI platforms will offer more accurate AI-powered solutions to improve business performance. To achieve this, research on Explainable AI, transfer learning, and reinforcement learning is still ongoing.
- Advances in tools that enable AI model development: With new technologies like automated machine learning (AutoML), AI platforms can create new machine learning models automatically and continuously improve their performance without human intervention.
To learn more about the future of AI, feel free to read our in-depth guide.
With the increasing number of citizen data scientists, and increasing data availability, accessibility and ease-of-use of advanced analytical resources become critical. AI platforms are valuable resources for democratizing building and maintenance of ML models (i.e. offering solutions for handling the end-to-end machine learning development cycle). Without these platforms, companies would need to spend a significant share of resources on developing and maintaining machine learning models.
These platforms can be implemented in any situation where machine learning is involved. Some common use cases are include:
- Marketing analytics
- Prescriptive Sales
- Intelligent Call Routing
- Data Management & Monitoring
- Fraud Detection
Feel free to visit our AI use cases/applications article for 100+ examples.
By using AI platforms, businesses can create machine learning models with ease. For example, these are some of the common machine learning approaches that businesses rely on while using AI platforms:
- Neural Networks: Neural networks are a set of algorithms and mathematical models that aim to mimic the human brain. It performs a particular task without using explicit instructions, relying on patterns and inferences. To create successful neural network models, businesses should identify what they want to do and decide if their available data is reliable enough.
- Transfer Learning: AI platforms can be used as a tool for transfer learning instead of creating a new model from scratch. When there is not enough data or time to train data, transfer learning enables businesses to benefit from a previously used AI model for a different task.
- Explainable AI: The advances of AI technologies also require creating understandable models. With Explainable AI, businesses can generate self-explanatory models that help them understand how their AI algorithms work and why they come up with particular results.
- Reinforcement Learning: Rather than traditional learning, reinforcement learning doesn’t look for patterns to make predictions. It makes subsequent decisions to maximize its reward, and it learns by experience. AI platforms can also benefit from this technology while creating new algorithms or models.
These machine learning techniques can be combined with symbolic (i.e. human-readable) approaches to solve problems in various domains:
- Natural Language Processing (NLP): This technology helps businesses to process and evaluate large volumes of data with natural language understanding, natural language generation, and speech recognition.
- Computer Vision: Businesses can automate specific tasks that require visual perception as humans do. Computer vision tasks include methods for acquiring, processing, analyzing, and understanding digital images, and extraction of high-dimensional data from the real world. Object recognition, motion estimation, and image restoration are a few examples of this technology.
- Cloud Systems: A robust cloud infrastructure provides improved scalability and access to resources for the implementation of complex AI and machine learning solutions. Considering large amounts of data, businesses need to combine both AI and cloud to make full use of their advantages.