Synthetic Data Generator
Companies rely on data to build machine learning models which can make predictions and improve operational decisions +Show More
Products | Position | Customer satisfaction | |||
---|---|---|---|---|---|
|
Leader
|
Satisfactory
|
|
||
BizDataX makes data masking/data anonymization simple, by cloning production or extracting only a subset of data. And mask it on the way, achieving GDPR compliance easier.
Basis for EvaluationWe made these evaluations based on the following parameters; Customer satisfaction
Average rating
Market presence
Company's number of employees
50-100 employees
Company's social media followers
1k-2k followers
Company
Type of company
private
Founding year
2006
|
|||||
|
Leader
|
Satisfactory
|
|
||
Tonic mimics your production data to create safe, useful, de-identified data for QA, testing, and development. Tonic’s synthetic data platform equips developers with the data they need to build products effectively, while achieving compliance and security. With Tonic, teams shorten development cycles, eliminate cumbersome data pipeline overhead, and mathematically guarantee the privacy of their data. Founded in 2018 with offices in San Francisco and Atlanta, the company is pioneering enterprise tools for database subsetting, de-identification, and synthesis. Thousands of developers use data generated with Tonic on a daily basis to build their products faster in industries as wide ranging as healthcare, financial services, logistics, edtech, and e-commerce. Working with customers like eBay, Flexport, and PwC, Tonic innovates to advance their goal of advocating for the privacy of individuals while enabling companies to do their best work. Build more in half the time with secure, useful data that moves as fast as your developers.
Basis for EvaluationWe made these evaluations based on the following parameters; Customer satisfaction
Average rating
Market presence
Company's number of employees
50-100 employees
Company's social media followers
5k-10k followers
Total funding
$10-50m
# of funding rounds
3
Latest funding date
September 29, 2021
Last funding amount
$10-50m
Company
Type of company
private
Founding year
2018
|
|||||
|
Leader
|
Satisfactory
|
|
||
GenRocket is the technology leader in synthetic data generation for quality engineering and machine learning use cases. We call it Synthetic Test Data Automation (TDA) and it's the next generation of Test Data Management (TDM). GenRocket provides a comprehensive self-service platform to more than 50 of the world's largest organizations who demand superior quality and efficiency in their quality engineering and data science operations. KEY FEATURES SPEED: Data generated at 10,000 rows/second and one billion rows in under two hours QUALITY: Any volume and variety of data (unique, negative, conditioned, permutations) REUSABILITY: Test Data Cases and Test Data Rules can be easily reused SELF-SERVICE: Model, design and deploy test data on-demand into CI/CD Pipelines SECURITY: Secure platform never uses or stores sensitive customer data VERSATILITY: 101+ data formats e.g. SQL, XML, JSON, EDI, PDF, Kafka, Parquet, AWS S3 VALUE FOR MONEY: Attractive license and implementation cost to maximizes value PROVEN BENEFITS ACCELERATION: 100 times faster than creating data in spreadsheets or via scripts COVERAGE: Improve test coverage from less than 50% to more than 90% to maximize quality VALUE: Reduce TCO by 90% when compared to traditional Test Data Management
Basis for EvaluationWe made these evaluations based on the following parameters; Customer satisfaction
Average rating
Market presence
Number of case studies
5-10 case studies
Company's number of employees
20-30 employees
Company's social media followers
400-1k followers
Company
Type of company
private
Founding year
2012
|
|||||
|
Leader
|
N/A
|
|
||
YData provides a data-centric platform that accelerates the development and increases the RoI of AI solutions by improving the quality of training datasets. Data scientists can now use automated data quality profiling and improve datasets by leveraging state-of-the-art synthetic data generation.
Basis for EvaluationWe made these evaluations based on the following parameters; Customer satisfaction
Average rating
Market presence
Company's number of employees
30-40 employees
Company's social media followers
5k-10k followers
Total funding
$1-5m
# of funding rounds
5
Latest funding date
October 11, 2021
Last funding amount
$1-5m
Company
Type of company
private
Founding year
2019
|
|||||
|
Leader
|
N/A
|
|
||
We help you discover, create, and subset test data; visualize test data coverage; and protect data so you can focus on development.
Basis for EvaluationWe made these evaluations based on the following parameters; Customer satisfaction
Average rating
Market presence
Company's number of employees
5k-10k employees
Company's social media followers
100k-1m followers
Company
Type of company
private
Founding year
1993
|
|||||
|
Challenger
|
N/A
|
|
||
Basis for EvaluationWe made these evaluations based on the following parameters; Customer satisfaction
Average rating
Market presence
Number of case studies
1-5 case studies
Company's number of employees
100-200 employees
Company's social media followers
4k-5k followers
Total funding
$100-250m
# of funding rounds
4
Latest funding date
March 1, 2022
Last funding amount
$50-100m
Company
Type of company
private
Founding year
2016
|
|||||
|
Challenger
|
N/A
|
|
||
Edgecase.ai is a data factory helping Fortune 500's and Startups alike in data annotation and generation of Ai training images and videos on our proprietary platform. Edgecase.ai helps solve the fundamental need of providing at scale data labeling to train the world's most advanced Ai vision and video recognition algorithms as well as AI agents in the fields of: Security, Retail, Healthcare, Agriculture, Industry 4.0 and the like.
info@edgecase.ai
Basis for EvaluationWe made these evaluations based on the following parameters; Customer satisfaction
Average rating
Market presence
Company's number of employees
1-5 employees
Company's social media followers
400-1k followers
# of funding rounds
2
Latest funding date
June 1, 2019
Company
Type of company
private
Founding year
2017
|
|||||
|
Challenger
|
N/A
|
|
||
CVEDIA is an AI solutions company that develops off the shelf computer vision algorithms using synthetic data - coined "synthetic algorithms". CVEDIA algorithms are ready to be deployed through 10+ hardware, cloud, and network options. CVEDIA technology is based off of their proprietary simulation engine, SynCity, and developed using data science and deep learning theory. The company operates cross-industry in infrastructure, security, smart cities, utilities, manufacturing, and aerospace.
Basis for EvaluationWe made these evaluations based on the following parameters; Customer satisfactionMarket presence
Number of case studies
1-5 case studies
Company's number of employees
10-20 employees
Company's social media followers
3k-4k followers
# of funding rounds
1
Latest funding date
August 28, 2018
Company
Type of company
private
Founding year
2016
|
|||||
|
Challenger
|
N/A
|
|
||
Neuromation is a Synthetic Data space building an AI Developer platform to build better models.
Basis for EvaluationWe made these evaluations based on the following parameters; Customer satisfactionMarket presence
Company's social media followers
5k-10k followers
|
|||||
|
Challenger
|
N/A
|
|
||
Hazy differentiates from the competition by offering models capable of generating high quality synthetic data with a differential privacy mechanism. Data can be tabular, sequential (containing time-dependent events, like bank transactions) or dispersed through several tables in a relational database.
Basis for EvaluationWe made these evaluations based on the following parameters; Customer satisfaction
Average rating
Market presence
Number of case studies
1-5 case studies
Company's number of employees
20-30 employees
Company's social media followers
2k-3k followers
Total funding
$10-50m
# of funding rounds
6
Latest funding date
March 28, 2023
Last funding amount
$5-10m
Company
Type of company
private
Founding year
2017
|
“-”: AIMultiple team has not yet verified that vendor provides the specified feature. AIMultiple team focuses on feature verification for top 10 vendors.
Sources
AIMultiple uses these data sources for ranking solutions and awarding badges in synthetic data generators:
Synthetic data Leaders
According to the weighted combination of 4 metrics
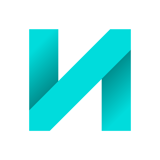
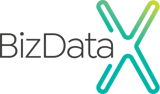
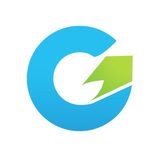
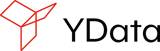
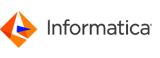
What are synthetic data
customer satisfaction leaders?
Taking into account the latest metrics outlined below, these are the current synthetic data customer satisfaction leaders:
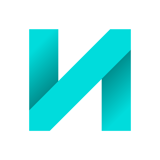
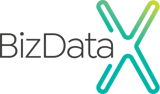
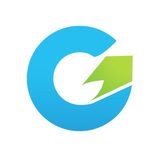
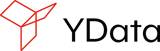
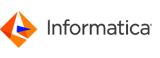
Which synthetic data solution provides the most customer satisfaction?
AIMultiple uses product and service reviews from multiple review platforms in determining customer satisfaction.
While deciding a product's level of customer satisfaction, AIMultiple takes into account its number of reviews, how reviewers rate it and the recency of reviews.
- Number of reviews is important because it is easier to get a small number of high ratings than a high number of them.
- Recency is important as products are always evolving.
- Reviews older than 5 years are not taken into consideration
- older than 12 months have reduced impact in average ratings in line with their date of publishing.
What are synthetic data
market leaders?
Taking into account the latest metrics outlined below, these are the current synthetic data market leaders:
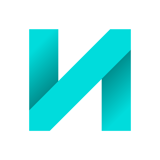
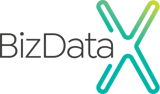
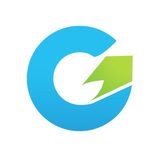
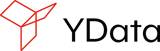
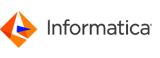
Which one has collected the most reviews?
AIMultiple uses multiple datapoints in identifying market leaders:
- Product line revenue (when available)
- Number of reviews
- Number of case studies
- Number and experience of employees
- Social media presence and engagement
What are the most mature synthetic data generators?
Which one has the most employees?
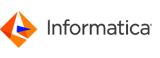
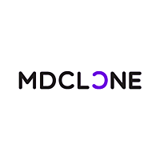
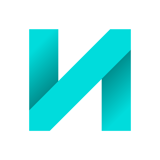
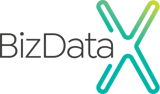

Which synthetic data companies have the most employees?
32 employees work for a typical company in this solution category which is 9 more than the number of employees for a typical company in the average solution category.
In most cases, companies need at least 10 employees to serve other businesses with a proven tech product or service. 11 companies with >10 employees are offering synthetic data generators. Top 3 products are developed by companies with a total of 5k employees. The largest company in this domain is Informatica with more than 5,000 employees. Informatica provides the synthetic data solution: Informatica Test Data Management Tool
Insights
What are the most common words describing synthetic data generators?
This data is collected from customer reviews for all synthetic data companies. The most positive word describing synthetic data generators is “Easy to use” that is used in 12% of the reviews. The most negative one is “Difficult” with which is used in 3% of all the synthetic data reviews.
What is the average customer size?
According to customer reviews, most common company size for synthetic data customers is 1-50 Employees. Customers with 1-50 Employees make up 44% of synthetic data customers. For an average Data solution, customers with 1-50 Employees make up 39% of total customers.
Customer Evaluation
These scores are the average scores collected from customer reviews for all synthetic data generators. Synthetic Data Generators are most positively evaluated in terms of "Customer Service" but falls behind in "Ease of Use".
Where are synthetic data vendors' HQs located?
Trends
What is the level of interest in synthetic data generators?
This category was searched on average for 5k times per month on search engines in 2024. This number has decreased to 0 in 2025. If we compare with other data solutions, a typical solution was searched 725 times in 2024 and this decreased to 0 in 2025.
Learn more about Synthetic Data Generators
There are 2 categories of approaches to synthetic data: modelling the observed data or modelling the real world phenomenon that outputs the observed data.
Modelling the observed data starts with automatically or manually identifying the relationships between different variables (e.g. education and wealth of customers) in the dataset. Based on these relationships, new data can be synthesized.
Simulation(i.e. Modelling the real world phenomenon) requires a strong understanding of the input output relationship in the real world phenomenon. A good example is self-driving cars: While we know the physical mechanics of driving and we can evaluate driving outcomes (e.g. time to destination, accidents), we still have not built machines that can drive like humans. As a result, we can feed data into simulation and generate synthetic data.
As expected, synthetic data can only be created in situations where the system or researcher can make inferences about the underlying data or process. Generating synthetic data on a domain where data is limited and relations between variables is unknown is likely to lead to a garbage in, garbage out situation and not create additional value.
Synthetic data enables data-driven, operational decision making in areas where it is not possible.
Any business function leveraging machine learning that is facing data availability issues can get benefit from synthetic data.
Any company leveraging machine learning that is facing data availability issues can get benefit from synthetic data.
Synthetic data is especially useful for emerging companies that lack a wide customer base and therefore significant amounts of market data. They can rely on synthetic data vendors to build better models than they can build with the available data they have. With better models, they can serve their customers like the established companies in the industry and grow their business.
Major use cases include:
- self driving cars
- customer level data in industries like telecom and retail
- clinical data
Increasing reliance on deep learning and concerns regarding personal data create strong momentum for the industry. However, deep learning is not the only machine learning approach and humans are able to learn from much fewer observations than humans. Improved algorithms for learning from fewer instances can reduce the importance of synthetic data.
Synthetic data companies can create domain specific monopolies. In areas where data is distributed among numerous sources and where data is not deemed as critical by its owners, synthetic data companies can aggregate data, identify its properties and build a synthetic data business where competition will be scarce. Since quality of synthetic data also relies on the volume of data collected, a company can find itself in a positive feedback loop. As it aggregates more data, its synthetic data becomes more valuable, helping it bring in more customers, leading to more revenues and data.
Access to data and machine learning talent are key for synthetic data companies. While machine learning talent can be hired by companies with sufficient funding, exclusive access to data can be an enduring source of competitive advantage for synthetic data companies. To achieve this, synthetic data companies aim to work with a large number of customers and get the right to use their learnings from customer data in their models.
Please note that this does not involve storing data of their customers. Synthetic data companies build machine learning models to identify the important relationships in their customers' data so they can generate synthetic data. If their customers gives them the permission to store these models, then those models are as useful as having access to the underlying data until better models are built.
Synthetic data is any data that is not obtained by direct measurement. McGraw-Hill Dictionary of Scientific and Technical Terms provides a longer description: "any production data applicable to a given situation that are not obtained by direct measurement".
Synthetic data allow companies to build machine learning models and run simulations in situations where either
- data from observations is not available in the desired amount or
- the company does not have the right to legally use the data. For example, GDPR "General Data Protection Regulation" can lead to such limitations.
Specific integrations for are hard to define in synthetic data. Synthetic data companies need to be able to process data in various formats so they can have input data. Additionally, they need to have real time integration to their customers' systems if customers require real time data anonymization.
For deep learning, even in the best case, synthetic data can only be as good as observed data. Therefore, synthetic data should not be used in cases where observed data is not available.
Synthetic data can not be better than observed data since it is derived from a limited set of observed data. Any biases in observed data will be present in synthetic data and furthermore synthetic data generation process can introduce new biases to the data.
It is also important to use synthetic data for the specific machine learning application it was built for. It is not possible to generate a single set of synthetic data that is representative for any machine learning application. For example, this paper demonstrates that a leading clinical synthetic data generator, Synthea, produces data that is not representative in terms of complications after hip/knee replacement.
While computer scientists started developing methods for synthetic data in 1990s, synthetic data has become commercially important with the widespread commercialization of deep learning. Deep learning is data hungry and data availability is the biggest bottleneck in deep learning today, increasing the importance of synthetic data.
Deep learning has 3 non-labor related inputs: computing power, algorithms and data. Machine learning models have become embedded in commercial applications at an increasing rate in 2010s due to the falling costs of computing power, increasing availability of data and algorithms.
Figure:PassMark Software built a GPU benchmark with higher scores denoting higher performance. Figure includes GPU performance per dollar which is increasing over time
While algorithms and computing power are not domain specific and therefore available for all machine learning applications, data is unfortunately domain specific (e.g. you can not use customer purchasing behavior to label images). This makes data the bottleneck in machine learning.
Deep learning relies on large amounts of data and synthetic data enables machine learning where data is not available in the desired amounts and prohibitely expensive to generate by observation.
While data availability has increased in most domains, companies face a chicken and egg situation in domains like self-driving cars where data on the interaction of computer systems and the real world is scarce. Companies like Waymo solve this situation by having their algorithms drive billions of miles of simulated road conditions.
In other cases, a company may not have the right to process data for marketing purposes, for example in the case of personal data. Companies historically got around this by segmenting customers into granular sub-segments which can be analyzed. Some telecom companies were even calling groups of 2 as segments and using them to predict customer behaviour. However, General Data Protection Regulation (GDPR) has severely curtailed company's ability to use personal data without explicit customer permission. As a result, companies rely on synthetic data which follows all the relevant statistical properties of observed data without having any personally identifiable information. This allow companies to run detailed simulations and observe results at the level of a single user without relying on individual data.
Observed data is the most important alternative to synthetic data. Instead of relying on synthetic data, companies can work with other companies in their industry or data providers. Another alternative is to observe the data.
The only synthetic data specific factor to evaluate for a synthetic data vendor is the quality of the synthetic data. It is recommended to have a through PoC with leading vendors to analyze their synthetic data and use it in machine learning PoC applications and assess its usefulness.
Typical procurement best practices should be followed as usual to enable sustainability, price competitiveness and effectiveness of the solution to be deployed.
Wikipedia categorizes synthetic data as a subset of data anonymization. This is true only in the most generic sense of the term data anonimization. For example, companies like Waymo use synthetic data in simulations for self-driving cars. In this case, a computer simulation involves modelling all relevant aspects of driving and having a self-driving car software take control of the car in simulation to have more driving experience. While this indeed creates anonymized data, it can hardly be called data anonymization because the newly generated data is not directly based on observed data. It is only based on a simulation which was built using both programmer's logic and real life observations of driving.